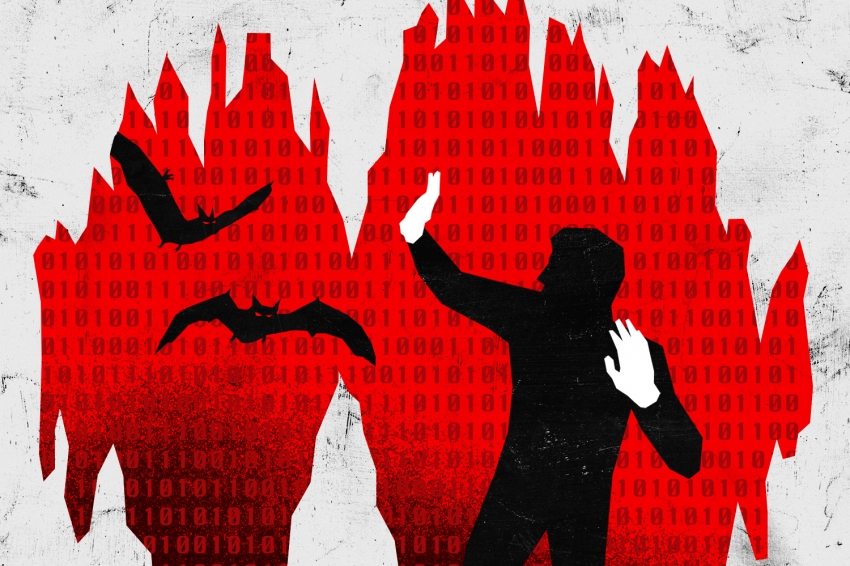
Computational methods repeatedly come up short.
By Nan Z. Da March 27, 2019Nan Z. Da is an assistant professor of English at the University of Notre Dame.

Quantitative methods are ascendant in literary studies, abetted by disproportionate funding, the absence of strict evaluative protocols, and a scarcity of knowledgeable and disinterested peer reviewers. It is time for the profession to take a closer look. Computational literary studies (CLS for short) — the most prominent strand of the digital humanities — applies computational methods to literary interpretation, from a single book to tens of thousands of texts. This usually entails feeding bodies of text into computer programs to yield quantitative results, which are then used to make arguments about literary form, style, content, or history. We are told, for instance, that digital analysis of 50,000 texts proves that there are “six or sometimes seven” basic literary plot types.
The Digital Humanities Wars
In the last six months, a new front has opened in the often fiery disciplinary disputes over the role of quantitative methods in the humanities. The University of Chicago Press published two major new works of computational literary scholarship, Andrew Piper’s Enumerations: Data and Literary Study and Ted Underwood’s Distant Horizons: Digital Evidence and Literary Change. And this month Nan Z. Da harshly criticized what she called “computational literary studies” in the pages of Critical Inquiry. This weekThe Chronicle Review is featuring essays by Underwood and Da, arguing from either side of the conflict. We’re also resurfacing some previous salvos in this war from our archive. — The EditorsDear Humanists: Fear Not the Digital Revolution by Ted UnderwoodThe Digital-Humanities Bust by Timothy BrennanWhat the Digital Humanities Can’t Do by Kathryn ConradBig-Data Doubts by Emma UprichardThe Humanities, Done Digitally by Kathleen Fitzpatrick
Not only has this branch of the digital humanities generated bad literary criticism, but it tends to lack quantitative rigor. Its findings are either banal or, if interesting, not statistically robust. The problem appears to be structural. In order to produce nuanced and sophisticated literary criticism, CLS must interpret statistical analysis against its true purpose; conversely, to stay true to the capacities of quantitative analysis, practitioners of CLS must treat literary data in vastly reductive ways, ignoring everything we know about interpretation, culture, and history. Literary objects are too few, and too complex, to respond interestingly to computational interpretation — not mathematically complex, but complex with respect to meaning, which is in turn activated by the quality of thought, experience, and writing that attends it.
Computational textual analysis itself is an imprecise science prone to errors. The degree to which this imprecision is acceptable depends on the size of your corpus and on the nature of your goals. In many sectors — but not in literary studies — machine-assisted textual analysis works. In such areas as social-media monitoring, biomedical research, legal discovery, and ad placement, unimaginably large sets of textual data are generated every second. Processing this data through computational and statistical tools is uncannily efficient at discovering useful and practical insights. CLS’s methods are similar to those used in professional sectors, but they can offer no plausible justification for their imprecision and drastic reduction of argumentative complexity. Results are often driven by completely prosaic explanations or choices baked into the method. The results of statistical tools are interpreted contrary to those tools’ true purposes or used decoratively; predictive models either do not have much explanatory power or else fail with the slightest, most reasonable adjustments. In “The Computational Case against Computational Literary Analysis,” recently published in Critical Inquiry, I go over examples of these mistakes in detail, and explain why and where they tend to occur.
CLS is not only a method but an evolving set of rhetorical strategies. The absence of true quantitative insights is excused through language drawn from the humanities. Practitioners of CLS shore up their shaky findings by analogizing them to familiar methods of traditional literary criticism. Meanwhile, they self-servingly define whatever is not quantitative literary analysis — close reading and theoretical interpretation, for example — as incomplete if not supplemented by some computationally enabled “distant reading” (in Franco Moretti’s famous phrase). CLS’s weakness as literary criticism is in turn excused with the language of exploratory data analysis. Under the cloak of interdisciplinarity, CLS has been able to frame weak findings as provisional, a demonstration of the need for more research. All of these tactics help to keep the enterprise going.
Alot of what is published in CLS is simply data-mining, a show-and-tell of quantitative findings matched without real conviction or commitment to existing bodies of knowledge. Such work is regarded suspiciously across disciplines, but those in the humanities are less likely to recognize it for what it is. The appeal to the discovery of patterns beyond our ken usually serves as a tell. In his most recent book, Distant Horizons (University of Chicago Press, 2019), Ted Underwood makes a case for computer-assisted literary discoveries on the grounds that “longer arcs of change have been hidden from us by their sheer scale — just as you can drive across a continent noticing mountains and political boundaries but never the curvature of the earth. A single pair of eyes at ground level can’t grasp the curve of the horizon, and arguments limited by a single reader’s memory can’t reveal the largest patterns organizing literary history.”Computational literary studies offers correctives to problems that literary scholarship was never confused about in the first place.
Here’s the thing about data: There will always be patterns and trends. Even a novel composed of pure gibberish, or a made-up literary history written by computers, will have naturally occurring statistically significant patterns. The promise of patterns alone is meaningless. Once you detect a pattern, you must subject it to a series of rigorous tests. Then you might have a meaningful pattern — although proving that the pattern persists and is meaningful involves many stages of technical assessment. Instead, what is seen in CLS is often undermotivated: There are loads of explanations, here is one more; or, my model has lots of predictions for all kinds of scenarios, let me go down the list and report the one that fits the data at hand.
In Distant Horizons, for example, Underwood asks the question: Isn’t it interesting that gender becomes more blurred after 1840? No one would say no. But what is really happening? The author and his collaborators claim to have come up with 15 models for predicting the gender of characters. These models are trained on 1,600 “characters” from each period, which are simply pronouns and proper names, using a natural-language processor that picks out the keywords associated with a particular character in textual samples of 54 words per decade. This model can correctly predict the gender of a character only 64 to 77 percent of the time, a predictive weakness that is then reframed as a trend: Gender boundaries are getting more blurred because our model becomes increasingly less predictive as it approaches the year 2007.
An experiment like this one wants to say something about the gender norms of fictional characters, but there is nothing here that necessarily has to do with fictional characters and their gender. Perhaps the ways people were described were becoming less gender-stereotypical in all genres of writing from 1840 to 2007, and you’re just seeing the fictional or novelistic manifestation of this progressive trend, which in and of itself really ought to surprise no one. More likely: Narrative styles and genres of fictional writing have become less homogenous over time (this is not hard to imagine), and so necessarily character “traits” are introduced in a text in an increasingly less homogenous manner. This could be true for any arbitrary trait: young/old, tall/short, characters whose names start with A versus characters whose names start with B. Your model for predicting the youth or agedness of a character would be progressively less accurate as you get closer to the present time. To show anything about gender in fiction, you’d have to rule out all of these possibilities — but then you’d be approaching the upper threshold of practicality. And why even do it, considering that the model’s definition of character and gender is so crude to begin with?
As for CLS’s claim to macroanalysis or “distant reading”: Perhaps no human being has read 300,000 or 10,000 books, but together we have, many times over, and have drawn careful summaries, generalizations, abstractions, and conclusions about them. And even if human beings have not read the entirety of literary history, neither have computational critics — not even close. Look closely, and you’ll discover what “covering” large corpora actually means for computational literary critics. A researcher picks out a few hundred short passages, looks at a small percentage of the most frequently occurring words, looks at a small percentage of overlapping words, and then calculates distances (which just means overlapping frequently-used-word similarity) between texts so that the difference between one novel and another becomes a single number, with even most of those data culled for the sake of representational convenience. Demystify the methodology, and it becomes clear that the vast majority of text is entirely unaccounted for. There’s a reason the quantitative study of literature returns again and again to what it isolates as dimorphic traits, like the somewhat ideologically suspect pairs black/white or male/female, or else to time-variance arguments (how something does or doesn’t change over time). Even if you do manage to train a computer to detect literary phenomena that come in entirely different forms and lengths, varying vastly in content, you still have too few of them for any kind of aggregate analysis.
Disciplinary judgment is what matters in the end. Literature itself, of course, does not resist quantification. Literature is made of letters, characters, and sounds, and so it can by definition be treated as measurable discrete units. Context can be recast as a concordance of proximate words; valence can be recast as a “similarity map.” Character, influence, genre, and so on can all be reduced to simple measures. Definitionally, you can measure anything in textual form — with a measurement error. The question is whether that error matters. You can believe that algorithms can learn structures in language, and also know that the detection of some of these structures can be found only with such a large measurement error that manual verification or qualification would be required in every single case. The point is that it is extremely hard, if not impossible, to train an algorithm to recognize a metaphor or accurately parse a line of poetry. These tasks involve problems in computer science and computational linguistics that approach the upper bounds of difficulty in those disciplines — but still approach only the lower bounds of difficulty in literary studies.
For example, modeling literary character through machine learning is extremely taxing from the perspective of computer science. The authors of a paper promising to “consider the problem of automatically inferring latent character types in a collection of 15,099 English novels” do a lot of work to improve the accuracy of clusters of words associated with characters created by particular authors, but, looking at their findings, you can see why literary critics would not be impressed:

Underneath CLS’s rhetoric of friendly collaboration is the entrenched belief that literary critics have no warrant for moving from specific examples to larger explanatory paradigms. As Andrew Piper, a leading digital humanist, says, “Until recently, we have had no way of testing our insights across a broader collection of texts, to move from observations about individual novels to arguments about things like the novel.”
It is ironic that practitioners of quantitative methods in literary studies accuse traditional literary critics of “often mov[ing] rapidly to draw broad cultural conclusions from scattered traces,” as Ted Underwood, David Bamman, and Sabrina Leeput it. In fact, it is computational critics who, as a matter of necessity, make such evidentiarily insufficient inferences, usually by misapplied analogies between some feature of the data and concepts in literary criticism or theory. CLS routinely relies on these concepts to provide plausible explanations or theoretical motivations for results that are nothing more than a description of the data. In their project on The Sorrows of Young Werther, for example, Andrew Piper and Mark Algee-Hewitt compared a standard visualization of the repetition of 91 words in Goethe’s oeuvre with theoretical paradigms as different as those of Gilles Deleuze, Alain Badiou, Bruno Latour, and Michel Foucault.
There is also the tendentious redescription of statistical tools. Michael Gavin, for instance, has recently arguedfor an affinity between William Empson’s notion of “ambiguity” — an elemental concept in literary theory — and vector space models, a way of mathematically measuring the similarity between texts. But Empson’s insight into ambiguity as a logical situation — when you cognitively cannot ascertain what has happened, or what meanings ought to be held together — is literally not the same as the word-sense disambiguation afforded by vector space models that is used in search engines and other forms of information retrieval. Polysemy (language’s capacity to mean many things at once) in Shakespeare is literally not, as the article suggests, analogous to a matrix of the most frequently occurring 5,000 words and their frequencies across 16 of Shakespeare’s plays. No amount of elegant argument can change those facts.Doubts about the value of quantitative work have nothing to do with anxieties about size, technology, or novelty.
The most misleading rhetoric occurs in CLS’s repacking of concepts and practices foundational to literary studies. Through the drastic narrowing of the meaning of literary-critical terms such as “keywords” and “close reading,” CLS offers correctives to problems that literary scholarship was never confused about in the first place. The computational paradigm of “distant reading” is itself a literalization drawn from “close reading,” a term that has served the discipline well as a description of smart, attentive, original exegesis. For CLS, “close reading” (which it claims to embrace) has been reduced to earnest but unilluminating combing of the text, word for word. Using this definition, CLS turns “close reading” into something that must be paired with “distant reading” to achieve completeness and broad explanatory power.
Literary interpretations and analyses that are based on smart, insightful, attentive, and original readings do not need supplementation by quantitative models. Uninspired readings — be they close, historicist, theoretical, or formal — will not be helped by superficial, number-crunched versions of the same. Literary studies has always offered large explanatory paradigms for moving from local observations to global ones; computationally assisted distant reading has no inherent claim on scale.
If you study literature with quantitative analysis, the results of your inquiry must satisfy the evaluative norms of both literary studies and the quantitative sciences. And yet CLS casts limitations that in the quantitative sciences would effectively end a line of inquiry as the philosophical outcome of the exercise. Serious criticism of the kind that should invalidate the work gets turned into philosophical meditations or platitudes about its ability to generate debate. At the conclusion of many published CLS papers, we often learn that a weak or nonexistent result is “exploratory,” “still in its early stages,” that the jury is still out. Exploration is perfectly valid, but it needs to take place before publication. Or, if one wishes to publish exploratory data analysis, it should be presented to disciplines that have better ways to evaluate the validity of the exercise. Otherwise, this kind of rhetoric guarantees that general errors, faulty reasoning, and failures in methodology and modeling will still merit publication, because they have improved techniques and provoked future inquiry, effectively reducing scholarship to an ongoing grant application.
RELATED CONTENT
- Dear Humanists: Fear Not the Digital Revolution
- The Digital-Humanities Bust PREMIUM
- What the Digital Humanities Can’t Do PREMIUM
- Big Doubts About Big Data
- The Humanities, Done Digitally
The messianic rhetoric of CLS, rooted in misapplied analogies between its methods and the core concepts of traditional literary study, has real consequences for the field: Resources unimaginable in any other part of the humanities are being redirected toward it, and things like positions, hiring and promotion, publishing opportunities, and grant money are all affected. But the important point is not inequity caused by allocation of resources away from so-called traditional humanistic inquiry but the quality of the work itself.
To be clear: What CLS needs is editorial stringency. Peer review ought to involve disinterested parties from both of the disciplines that CLS straddles. Scripts and data work should be presented at the time of submission. Replication is difficult, and much ink has been spilled on this topic, but there must be some agreement on sensible guidelines for review. More crucially, in assessing CLS work we must look past the analogies, the bells and whistles, the smoke and mirrors, to see what really, literally, has been done — count by count — to prepare text for statistical inference. The art of analogy should be reserved for situations in which the primary contribution isn’t the mere description of the effects of using statistical tools that have very specific uses. Evaluating CLS means more than checking for robustness. It means starting with these questions: Has the work given us a concrete, and testable, channel for understanding the predictive mechanism that it has proposed? Does it ask an interesting question as literary criticism, and answer it without recourse to brute comparison?
Doubts about the value and validity of quantitative work in literary studies have nothing to do with anxieties about large quantities, technology, novelty, or futurity. Aside from actual grant proposals, scholarship should be evaluated on the condition that it exists and is good, not on the premise that it is a taste of better things to come. Those who insist on this sensible rule should not be subjected to patronizing accusations that they are reactionary or stodgy, closed off to exploration, discovery, interdisciplinarity, and pragmatic compromise.
Let us speak practically. The thing about literature is that there isn’t a lot of it, comparatively speaking. At some point (if it hasn’t happened already), genres will be classified and reclassified using word frequencies, “topics” extracted, “gender” predicted. We’ll have been shown at various levels of superficiality how much literary forms and styles have evolved over time while also staying the same. Variations on these kinds of studies are limitless but, then again, computers are fast. A consolidation of CLS’s findings and results will curb the rhetoric of perpetual novelty. It can’t publish at the rate that it does and still claim the coveted status of a thing yet to come. At some point, we will have tried out the “new” perspective and decided whether or not it was worth exploring. The time to make that decision is now.